Introduction
Businesses today face overwhelming amounts of data from various sources, yet struggle to extract meaningful insights. Without proper data analysis, organizations risk missed opportunities, inefficiencies, and misguided decision-making, putting them behind competitors. Learning the essentials of data analysis is key to unlocking valuable insights, enabling businesses to make informed and effective decisions that drive growth.
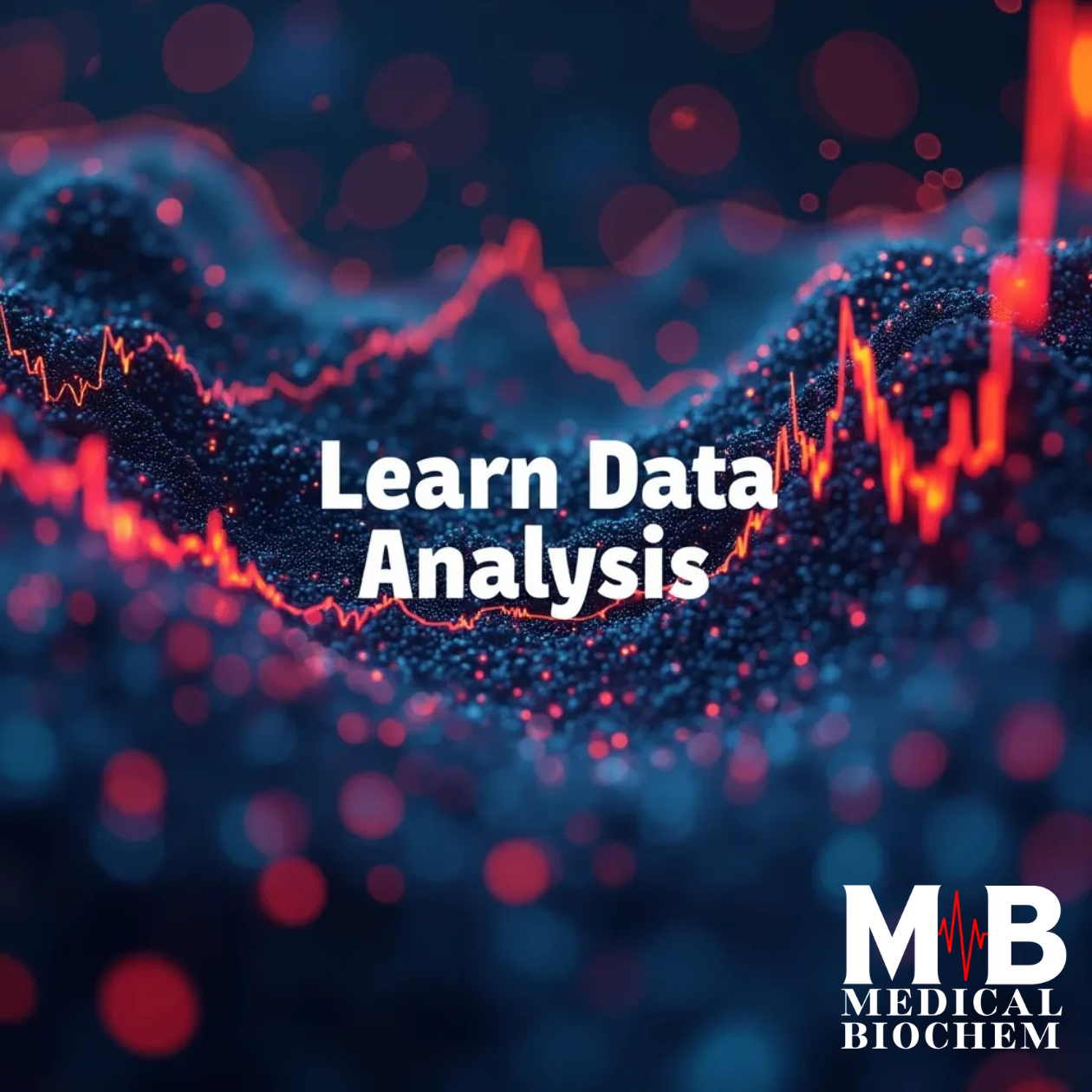
Understanding Data Analysis: What It Means and Why It Matters
What is Data Analysis?
Data analysis refers to the systematic examination of data to uncover useful insights, draw conclusions, and facilitate decision-making. It is not merely about collecting numbers and figures but transforming them into actionable information. Through analysis, patterns can be identified, trends can be forecasted, and valuable knowledge can be derived, making it a powerful tool for businesses across all industries.
The Importance of Data Analysis in Today’s Business Environment
- Informed Decision-Making: Data analysis helps organizations make decisions based on evidence rather than assumptions. By using reliable data, businesses can understand their strengths and weaknesses more clearly, leading to more informed strategies and plans.
- Operational Efficiency: Analyzing operational data allows for identifying inefficiencies and optimizing processes. If a business identifies a particular step in its supply chain that causes delays, it can take focused actions to resolve the bottleneck.
- Customer Insights: Understanding what customers want is essential for a business’s success. Analysis of data provides a window into customer preferences, behaviors, and needs, making it possible to offer personalized experiences that boost customer satisfaction and loyalty.
- Managing Risks and Predicting Trends: Businesses can use this to evaluate risks and identify market trends. By analyzing historical data, companies can predict upcoming trends and mitigate potential risks, providing an advantage in a competitive landscape.
Different Types of Data Analysis
The field of data analysis includes several approaches, each designed to answer different types of questions:
- Descriptive Analysis: This form of analysis provides a summary of historical data, revealing trends and past performance. Descriptive analysis might indicate that sales were highest during the holiday season or that user engagement peaked during a particular campaign.
- Diagnostic Analysis: While descriptive analysis explains what happened, diagnostic analysis aims to explain why it happened. If a business notices a drop in customer retention, diagnostic analysis would examine factors like changes in user experience or external competition to understand the reason.
- Predictive Analysis: Using historical data, predictive analysis seeks to predict future outcomes. This method often leverages machine learning and statistical models to anticipate trends, such as forecasting sales for the next quarter based on previous data.
- Prescriptive Analysis: This type of analysis goes beyond predictions by recommending actionable steps. For instance, if a retailer predicts a surge in demand for a product, prescriptive analysis can suggest increasing inventory to meet the projected demand.
Data Analysis Process
Data analysis is a step-by-step process that transforms raw data into actionable insights. The following are the key steps involved:
- Define Objectives: The initial step in the analysis is to define clear objectives. What is the specific problem or question that needs to be addressed? This helps in guiding the analysis process.
- Data Collection: Relevant data is gathered from various sources, such as customer databases, surveys, logs, and online platforms. The quality of data collected is vital since inaccurate data will lead to misleading results.
- Data Cleaning: Data cleaning is an essential step, involving the removal of duplicates, handling of missing values, and correction of inconsistencies. Clean data leads to more accurate analysis.
- Data Analysis: At this stage, statistical techniques and algorithms are applied to extract meaningful insights. Tools like Python, R, or SQL may be used to perform various analyses on the dataset, uncovering patterns and trends.
- Interpretation and Reporting: The final step is interpreting the results and presenting them in a format that is easy to understand. Data visualization tools such as Tableau and Power BI are effective in summarizing data through charts, graphs, and dashboards.
Tools Commonly Used for Data Analysis
- Excel: Excel remains a popular choice for simple data analysis due to its accessibility and ease of use. It is ideal for small datasets and preliminary data manipulation.
- Python and R: Python and R are programming languages favored for more complex analysis. They offer a wide variety of libraries and packages for statistical modeling, data manipulation, and visualization.
- SQL: SQL is essential for working with databases, especially when data needs to be extracted, updated, or managed in a structured manner. It’s an important tool for analysts who need to work with large databases.
- Tableau and Power BI: These data visualization tools provide a way to present data insights interactively. They transform complex data into visual stories, making it easier for stakeholders to understand key information.
Challenges Faced in Data Analysis
The increasing amount of data generated poses unique challenges for analysts:
- Volume and Variety of Data: Organizations generate a tremendous volume of data that comes in various forms—structured, semi-structured, and unstructured. Handling this diversity can be a major hurdle.
- Data Quality: Inaccurate or incomplete data often leads to flawed insights. Ensuring data quality through proper cleaning and validation is crucial for obtaining reliable results.
- Security and Privacy Concerns: Collecting and analyzing data requires careful handling of sensitive information to prevent breaches and comply with privacy laws. Mismanagement can lead to severe legal and reputational consequences.
The Impact of Data Analysis Across Industries
Data analysis is not limited to one specific sector; it influences a wide range of industries:
- Retailers: Retailers use this to understand customer buying habits, optimize inventory management, and deliver personalized marketing campaigns.
- Healthcare: Data science in healthcare helps predict disease outbreaks, optimize patient treatment plans, and improve overall patient care quality.
- Finance: In finance, it plays a crucial role in fraud detection, assessing risk levels, and predicting market trends to guide investments.
- Manufacturing: In manufacturing, it is used to monitor production processes, predict equipment failures, and ensure quality control.
Conclusion
Data analysis has become a critical component of the modern business landscape. It provides companies with the tools they need to make informed decisions, optimize processes, and respond to emerging market trends. Regardless of industry, analysis of data serves as a compass, guiding organizations toward success by providing clear and actionable insights. Now that you understand the fundamentals, it’s time to take the next step. Whether you’re an individual learning data skills or a business looking to leverage data for growth, analysis of data opens the door to endless opportunities.
If you’re ready to transform your data into actionable insights and make smarter decisions, get in touch with our site today! Learn how you can harness the power of data to drive your business forward.